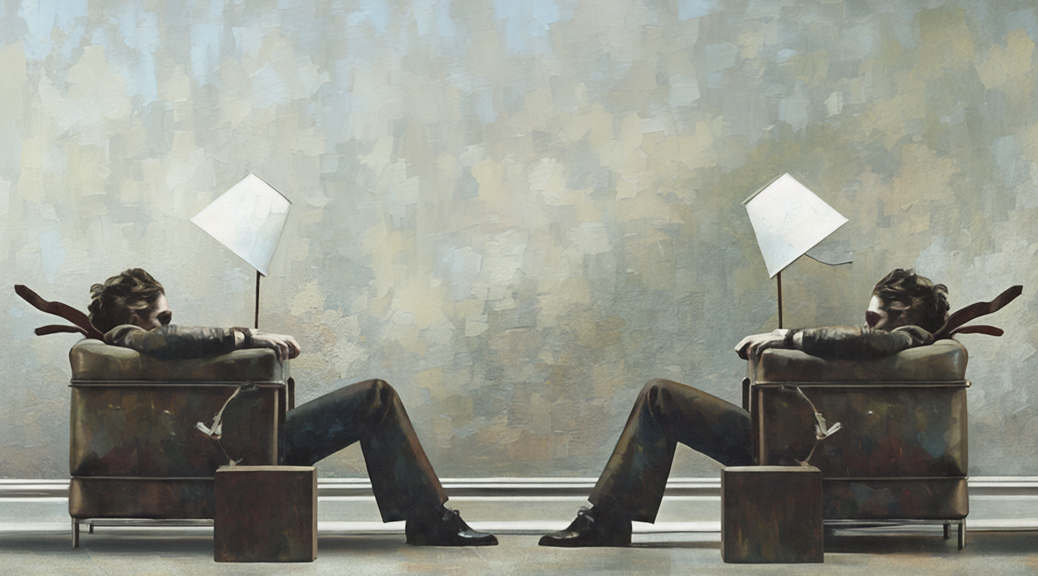
Is it Real or is it AI? Introducing Elai and the Future of Video Creation
This blog post is about AI, the future, Bill Gates, and – most importantly – an introduction to Elai – a new video creation tool coming to LUC soon!
AI and The Future
Differing reactions towards the ascent and ongoing advancement of AI capabilities have been well documented for years. Based on a survey from the Alan Turing Institute, the majority of participants are optimistic about AI usage in a variety of different sectors. In the article “The Age of AI Has Begun,” Bill Gates highlights several key areas where AI is making significant strides including healthcare, education, and climate change. He believes that AI can improve diagnostics, personalize learning experiences, and optimize resource management to combat environmental challenges.
In contrast with the optimism expressed in the sources above, AI certainly has its detractors and alarmists ranging from educators who worry about academic dishonesty to employees who panic at the prospect of unemployment. In the same article, Bill Gates also points out the ethical considerations and potential risks associated with AI, such as job displacement and the importance of ensuring that AI systems are aligned with human values.
On a much smaller scale than the stakes mentioned by Bill Gates, AI is already transforming the way we create videos for the better. Platforms such as Elai empower users with AI by enabling them to use their creative skills to produce high quality resources.
What is Elai?
Elai is an innovative platform that leverages artificial intelligence to revolutionize the way we create and manage video content. It empowers users to generate professional-quality videos in a matter of minutes, eliminating the complexities that often accompany traditional video production processes. With its user-friendly interface and advanced technology, Elai caters to a wide range of users, from marketers and educators to content creators and businesses looking to enhance their online presence.
At the core of Elai’s functionality is its AI-driven text-to-video technology. Users can simply input text scripts, and the platform automatically generates videos complete with dynamic visuals, voiceovers, and animations. This feature significantly reduces the time and resources needed for video production which makes it an invaluable tool for those who require quick turnarounds without compromising on quality. The ability to create videos in multiple languages further broadens its appeal, which allows users to reach diverse audiences around the globe.
One of the standout features of Elai is its extensive library of customizable templates. These templates are designed to cater to various industries and purposes. Users can choose from a variety of styles, colors, and layouts, enabling them to create videos that align with their brand identity. This level of customization ensures that the final product is not only professional but also uniquely tailored to the user’s needs.
Additionally, Elai offers a selection of AI-generated avatars that can serve as virtual presenters in videos. This feature allows for a more engaging and personal touch, as users can select avatars that resonate with their target audience. The avatars can express emotions and deliver the script in a natural manner to make the content more relatable and captivating.
The platform also includes editing tools that enable users to refine their videos easily. Users can trim clips, adjust timings, and insert additional elements such as images and graphics, all without requiring advanced video editing skills. This accessibility democratizes video production, allowing anyone, regardless of their technical expertise, to create high-quality content.
In conclusion, Elai stands at the forefront of video content creation by combining the power of AI with user-friendly design to simplify the video production process. Its diverse features including customizable templates, AI avatars, and collaborative tools make it an essential platform for anyone looking to create engaging, professional-quality videos quickly and efficiently.
How does AI enhance video production?
The integration of AI in video production significantly enhances creativity in several compelling ways:
- Automated Content Generation: AI tools can quickly transform text scripts into visually engaging videos. This automation allows creators to focus more on the creative aspects of storytelling rather than getting bogged down in technical details.
- Enhanced Customization and Personalization: AI enables users to customize videos easily, offering a wide range of templates, styles, and elements tailored to specific audiences or brand identities.
- Data-Driven Insights: AI can analyze audience engagement metrics and feedback, providing creators with valuable insights into what resonates with viewers. This data can inspire new ideas and empower creators to refine their content strategies and innovate based on real-time feedback.
- Collaborative Tools: Many AI video production platforms offer collaborative features that facilitate teamwork among creators.
- Rapid Prototyping: AI allows for quick iterations on video concepts. Creators can generate multiple versions of a video in a short time while experimenting with different scripts, visuals, and audio options.
- Accessibility for Non-Experts: AI democratizes video production, enabling individuals without extensive technical skills to create high-quality content.
- Inspiration Through AI Suggestions: AI can analyze existing content and suggest creative directions, themes, or styles based on trends and successful videos. These suggestions can spark inspiration by helping creators think outside the box and develop unique concepts.
In summary, the use of AI in video production enhances creativity by automating mundane tasks, providing customization options, offering data-driven insights, and enabling collaboration. This empowers creators to focus on their artistic vision and produce innovative, engaging content that resonates with audiences. Elai provides users with an effective enhancement to their workflow but without completing the entire task on their behalf. While Elai does not conjure presentations out of thin air, it gives users a helpful push in creating an excellent final product.
When will Elai be available at LUC?
Elai will be available at LUC on a trial basis for one calendar year beginning on May 1st, 2025. Stay tuned for more details on how to request access to this exciting pilot!